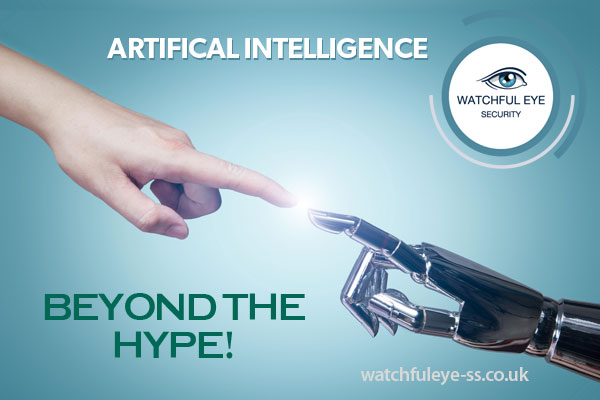
Artificial Intelligence and Loss Prevention: Seeing through the hype
Machine learning focuses on pattern recognition wherein the goal is to identify patterns within data that can be used to make predictions or otherwise solve problems. Two major types of machine learning are supervised learning and unsupervised learning.
Supervised learning can be thought of as ‘learning by example’. In supervised learning, there’s a known quantity that we’re trying to predict or a specific pattern that we’re striving to recognise. We may want to predict the risk of cashier fraud using behavioural and activity data. Assuming that we have enough examples of fraudulent behaviour, we can use AI to learn how to make these predictions.
Unsupervised learning applies when we don’t know exactly what we want to predict, but we have a lot of data that we’re trying to understand. Using unsupervised learning, we can cluster data into similar groups. We can also perform anomaly detection, finding patterns or events that don’t match what we’d expect to see.
Applied to customer or employee behaviour, this anomaly detection could potentially alert us to behaviours that might be worth further investigation. For example, if one employee is behaving significantly differently from all of the others, we can then investigate further to find the root cause.
Cognitive computing
Whereas machine learning deals with pattern recognition and learning to solve problems that humans cannot, cognitive computing tries to create systems that solve problems in the same way that humans would do. This includes things like computer vision, natural language processing (ie understanding meaning and sentiment behind text and spoken words) and interactive dialogue (ie chatbots).
Cognitive computing and machine learning often go hand-in-hand. For example, cognitive computing can be used to understand why a customer leaves an angry comment on social media about their store visit, while machine learning can be used to predict if the customer will ever return to the store. For its part, computer vision can be used to detect a human within a video. Machine learning may be employed to determine if that human is putting an item from the shelf under their jacket.
Knowing the difference
Knowing more about the specific types of AI and their goals can help in separating the hype from products that really leverage AI to complete advanced tasks. Something to keep in mind is that AI isn’t a magic bullet. Rather, it’s a tool to be applied to a specific problem.
It’s probably not enough for a company to claim that it “uses cutting-edge AI to enhance loss prevention” if that company cannot describe precisely what they’re using AI to predict or to interpret. A more credible claim would be to “predict the risk of cashier fraud using past behaviour”.
Be wary of broad generalities and make sure that you understand precisely what data is being used and what predictions are being made by AI. AI can be applied to loss prevention to greatly enhance the effectiveness and efficiency of such professionals, but it’s important to critically evaluate the claims being made in order to make sure you’re investing in actual AI and not just buying into the hype (of which there is quite a lot right now).
Protecting against AI hype
We’ve started to see some companies falsely market ‘Business Intelligence’ tools as AI tools. This is not because the tool has evolved, but rather because the market demand has changed from ‘Business Intelligence’ to AI. On that basis, they’ve simply rebranded their offering.
Unfortunately, the effort to move from a ‘Business Intelligence’ tool to an AI tool requires much more than simply relabelling the product. The big difference between AI and ‘Business Intelligence’ is the questions that are being answered. The latter is often used to address what has happened, whereas AI can be used to evaluate what’s going to happen next.